Bode Lecture
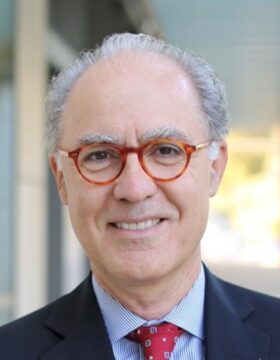
Miroslav Krstic
University of California San Diego, USA
Title: Machine Learning: Bane or Boon for Control?
Time: Friday, December 15, 08:30 – 09:30 (FrPr1)
Location: Roselle Simpor Main Ballroom 4601AB-4806
Abstract: Control theory is hardly alone among scientific communities experiencing some “obsolescence anxiety” in the face of machine learning, where decades - or centuries - of building first-principles models and designs are supplanted by data. While ML real-time feedback is unlikely to attain the adaptive control’s closed-loop guarantees for unstable plants that lack persistency of excitation, our community, adept at harnessing new ideas, has generated in a few years many other adroit ways to incorporate ML - from lightening methodological complexities to circumventing difficult constructions.
Rather than walking away from certificate-bearing control tools built by generations of control researchers, in this lecture I seek game-changing “supporting roles” for ML, in control implementation. I present the emerging subject of employing the latest breakthrough in deep learning approximations of not functions but function-to-function mappings (nonlinear operators) in the complex field of PDE control. With “neural operators,” entire PDE control methodologies are encoded into what amounts to a function evaluation, leading to a thousandfold speedup and enabling PDE control implementations. Deep neural operators, such as DeepONet, mathematically guaranteed to provide an arbitrarily close accuracy in rapidly computing control inputs, preserve the stabilization guarantees of the existing PDE backstepping controllers. Applications range from traffic and epidemiology to manufacturing, energy generation, and supply chains.
Biography: Miroslav Krstic is distinguished professor and founding director of the Cymer Center for Control Systems and Dynamics at UC San Diego, where he also serves as Senior Associate Vice Chancellor for Research. He is Fellow of IEEE, IFAC, SIAM, ASME, AAAS, IET (UK), AIAA (Assoc. Fellow), Serbian Academy of Sciences, and Academy of Engineering of Serbia. Besides the Bode Lecture Prize, he has received the Bellman Award, SIAM Reid Prize, ASME Oldenburger Medal, Nyquist Lecture Prize, Paynter Award, Ragazzini Education Award, IFAC Ruth Curtain Distributed Parameter Systems Award, IFAC Nonlinear Control Systems Award, IFAC Adaptive and Learning Systems Award, Chestnut Textbook Prize, Balakrishnan Award for the Mathematics of Systems, CSS Distinguished Member Award, and several early career and paper awards. He is currently Editor-in-Chief of Systems & Control Letters and Senior Editor in Automatica, having previously served as SE in IEEE TAC and CSS VP for Technical Activities. Krstic has coauthored books and papers on adaptive, nonlinear, and stochastic control, extremum seeking, control of PDE systems including turbulent flows, and control of delay systems. Industrial uses of his algorithms have been in photolithography for microchips, charged particle accelerators, oil drilling, spectroscopy on the Mars Rover Curiosity, Li-ion batteries, and arrestment of landing aircraft.
Plenary Talks
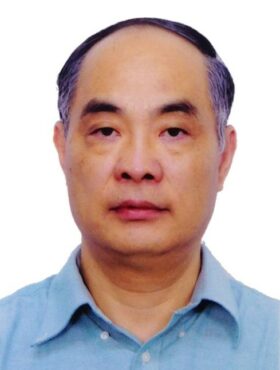
Jie Huang
The Chinese University of Hong Kong, China
Title: The Evolution of the Distributed Observer and Its Applications
Time: Wednesday, December 13, 08:30 – 09:30 (WeSP1)
Location: Melati Main 4001AB-4104
Abstract: A typical multi-agent system is composed of a follower system consisting of multiple subsystems called followers and a leader system whose output is to be tracked by the followers. What makes the control of a multi-agent system challenging is that the control law needs to be distributed in the sense that it must satisfy time-varying communication constraints. A special case of distributed control is where all the followers can access the information of the leader. For this special case, one can design, for each follower, a conventional control law based on the information of the leader. The collection of these conventional control laws constitutes the so-called purely decentralized control law for the multi-agent system. Nevertheless, the purely decentralized control law is not feasible due to the communication constraints. In this talk, we will introduce a framework for designing a distributed control law by cascading a purely decentralized control law and a so-called distributed observer for the leader system, which is a dynamic compensator that estimates and transmits the leader’s information to each follower over a communication network. Such a control law is called the distributed observer-based control law and has found its applications to such problems as consensus, synchronization, flocking, formation, and distributed Nash equilibrium seeking. The core of this design framework is the distributed observer for a linear leader system, which was initiated in 2010 for dealing with the cooperative output regulation problem, and has experienced three phases of developments. In the first phase, the distributed observer is only capable of estimating and transmitting the leader’s state to every follower assuming every follower knows the dynamics of the leader. In the second phase which started in 2015, the distributed observer is rendered the capability of estimating and transmitting not only the leader’s state but also the dynamics of the leader to every follower provided that the leader’s children know the information of the leader. Such a dynamic compensator is called an adaptive distributed observer for a known leader system. The distributed observer was further developed in 2017 for linear leader systems containing unknown parameters, thus entering its third phase of the development. Such a dynamic compensator is called an adaptive distributed observer for an unknown leader as it not only estimates the state but also the unknown parameters of the leader. We will start with an overview on the development of the distributed observer and then highlight the recent results on establishing an output-based adaptive distributed observer for an unknown leader system over jointly connected communication networks. Extensions, variants and applications of the distributed observer will also be touched.
Biography: Jie Huang studied Power Engineering at Fuzhou University from 1977 to 1979 and Circuits and Systems at Nanjing University of Science and Technology (NUST) from 1979 to 1982 for a Master degree. He completed his Ph.D. study in automatic control at Johns Hopkins University in 1990. After a year with Johns Hopkins University as a postdoctoral fellow and four years with industry in USA, he joined the Department of Mechanical and Automation Engineering, the Chinese University of Hong Kong (CUHK) in September 1995, and is now Choh-Ming Li Research Professor of Mechanical and Automation Engineering. He was a “State Specially Recruited Expert” of China, served as a Science Advisor to the Leisure and Cultural Services Department of Hong Kong Special Administrative Region, Honorary Advisor to Hong Kong Science Museum, and Chairman of the Department of Mechanical and Automation Engineering, CUHK. His research interests include nonlinear control, networked multi-agent systems control, game theory, and guidance and control of flight vehicles. He has authored/co-authored four monographs and over 400 papers.
Jie Huang has received several awards such as Outstanding Contribution Award by Technical Committee of Control Theory, China Association of Automation, in 2015, China State Natural Science Award, second prize, in 2010, Croucher Senior Research Fellowship award in 2006, and Changjiang Professor Award in 2002. He was elected HKIE Fellow in 2017, CAA Fellow in 2010, IFAC Fellow in 2009, and IEEE Fellow in 2005, and is now a life fellow of IEEE.
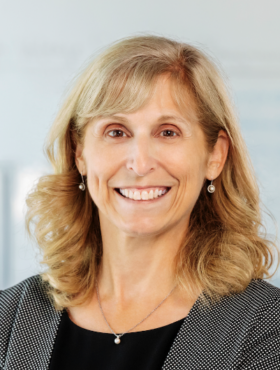
Naomi Ehrich Leonard
Princeton University, USA
Title: Fast and Flexible Multi-Agent Decision-Making
Time: Wednesday, December 13, 08:30 – 09:30 (WeSP2)
Location: Orchid Main 4202-4306
Abstract: A multi-agent system should be capable of fast and flexible decision-making if it is to successfully manage the uncertainty, variability, and dynamic change encountered when operating in the real world. Decision-making is fast if it breaks indecision as quickly as indecision becomes costly. This requires fast divergence away from indecision in addition to fast convergence to a decision. Decision-making is flexible if it adapts to signals important to successful operations, even if they are weak or rare. This requires tunable sensitivity to input for modulating regimes in which the system is ultra-sensitive and in which the system is robust. Nonlinearity and feedback in the multi-agent decision-making dynamics are necessary to meet these requirements.
I will present theoretical principles, analytical results, and applications of a general model of decentralized, multi-agent, and multi-option, nonlinear opinion dynamics that enables fast and flexible decision-making. I will explain how the critical features of fast and flexible multi-agent decision-making depend on nonlinearity, feedback, and the structure of the inter-agent communication network and a belief system network. And I will show how the theory and results provide a principled and systematic means for designing and analyzing multi-agent decision-making in systems ranging from multi-robot teams to social networks.
Biography: Naomi Ehrich Leonard is Chair and Edwin S. Wilsey Professor of Mechanical and Aerospace Engineering and associated faculty in Applied and Computational Mathematics at Princeton University. She is former Director of the Council on Science and Technology at Princeton and Founding Editor of the Annual Review of Control, Robotics, and Autonomous Systems. She received her BSE in Mechanical Engineering from Princeton University and her PhD in Electrical Engineering from the University of Maryland. She is a MacArthur Fellow, elected member of the American Academy of Arts and Sciences, and winner of the 2023 IEEE Control Systems Award and the 2017 IEEE CSS Henrik W. Bode Lecture Prize. Leonard is Fellow of IEEE, IFAC, SIAM, and ASME. Her current research focuses on dynamics, control, and learning for multi-agent systems on networks with application to multi-robot teams, collective animal behavior, social networks, and other multi-agent systems in technology, nature, and the visual and performing arts.
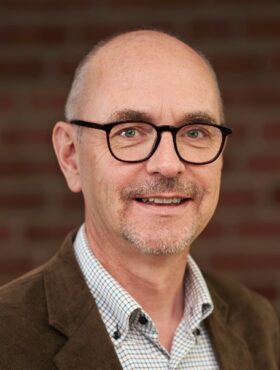
Anders Rantzer
Lund University, Sweden
Title: Dual Control Revisited
Time: Thursday, December 14, 08:30 – 09:30 (ThSP2)
Location: Orchid Main 4202-4306
Abstract: The term dual control was introduced in the 1960s to describe the tradeoff between short term control objectives and actions to promote learning. A closely related term is the exploration-exploitation tradeoff. This lecture will review some settings where dual controllers can be optimized efficiently, both for practical purposes and for a more fundamental understanding of the interplay between learning and control.
The starting point will be the standard setting of linear systems optmized with respect to quadratic cost. However much of modern learning theory is developed in a discrete setting. By investigating similarities and differences between the two frameworks, we will shed light on the dual control problem and discover new promising results and directions for research.
Biography: Anders Rantzer was appointed professor of Automatic Control at Lund University, Sweden, after a PhD at KTH Stockholm in 1991 and a postdoc 1992/93 at IMA, University of Minnesota. The academic year of 2004/05 he was visiting associate faculty member at Caltech and 2015/16 he was Taylor Family Distinguished Visiting Professor at University of Minnesota. Rantzer is a Fellow of IEEE, member of the Royal Swedish Academy of Engineering Sciences, Royal Physiographic Society in Lund and former chairman of the Swedish Scientific Council for Natural and Engineering Sciences. His research interests are in modeling, analysis and synthesis of control systems, with particular attention to scalability, adaptation and applications in energy networks.
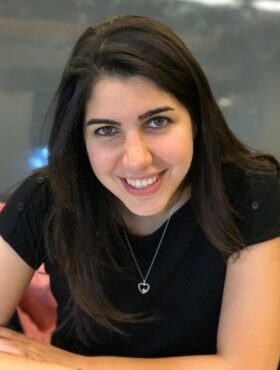
Dorsa Sadigh
Stanford University, USA
Title: Interactive Learning and Control in the Era of Large Models
Time: Thursday, December 14, 08:30 – 09:30 (ThSP1)
Location: Melati Main 4001AB-4104
Abstract:In this talk, I will discuss the problem of interactive learning by discussing how we can actively learn objective functions from human feedback capturing their preferences. I will then talk about how the value alignment and reward design problem can have solutions beyond active preference-based learning by tapping into the rich context available from large language models. In the second section of the talk, I will more generally talk about the role of large pretrained models in today’s robotics and control systems. Specifically, I will present two viewpoints: 1) pretraining large models for downstream robotics tasks, and 2) finding creative ways of tapping into the rich context of large models to enable more aligned embodied AI agents. For pretraining, I will introduce Voltron, a language-informed visual representation learning approach that leverages language to ground pretrained visual representations for robotics. For leveraging large models, I will talk about a few vignettes about how we can leverage LLMs and VLMs to learn human preferences, allow for grounded social reasoning, or enable teaching humans using corrective feedback. Finally, I will conclude the talk by discussing some preliminary results on how large models can be effective pattern machines that can identify patterns in a token invariant fashion and enable pattern transformation, extrapolation, and even show some evidence of pattern optimization for solving control problems.
Biography: Dorsa Sadigh is an assistant professor in Computer Science and Electrical Engineering at Stanford University. Her research interests lie in the intersection of robotics, learning, and control theory. Specifically, she is interested in developing algorithms for safe and adaptive human-robot and human-AI interaction. Dorsa received her doctoral degree in Electrical Engineering and Computer Sciences (EECS) from UC Berkeley in 2017, and received her bachelor’s degree in EECS from UC Berkeley in 2012. She is awarded the Sloan Fellowship, NSF CAREER, ONR Young Investigator Award, AFOSR Young Investigator Award, DARPA Young Faculty Award, Okawa Foundation Fellowship, MIT TR35, and the IEEE RAS Early Academic Career Award.